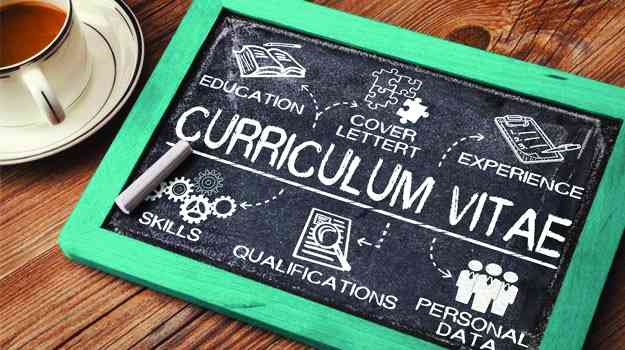
The curriculum vitae (CV) or resume stands as a cornerstone of modern personnel selection processes worldwide. Its use is pervasive; studies indicate that the vast majority of employers globally rely on analysing resumes and cover letters during hiring.
On average, over 80% of employers worldwide utilise CVs in their selection procedures, making it one of the most common selection tools after the interview.
This near-universal adoption stems largely from the perceived convenience and intuitive appeal of the CV. It appears to offer employers immediate access to a candidate's educational background, qualifications, work history, and skill set, seemingly providing a concise summary relevant to the job opening.
Understanding predictive validity
When it comes to personnel selection, the central measure of a tool's effectiveness is its predictive validity — the degree to which assessments derived from a selection tool accurately forecast an individual's future performance on relevant job criteria.
Predictive validity addresses the fundamental question: Does this measure predict who will perform well on the job? Several meta-analyses (statistical techniques that combine results from multiple studies) have examined whether CVs actually predict job performance, and the findings paint a complex picture.
Unstructured CV reviews: Challenges
When it comes to typical, unstructured CV screening — where recruiters subjectively review resumes without standardised criteria — the predictive validity is disappointingly low.
- Where are Zim universities getting it wrong?
- How African mass markets enable participants to write their own CVs
- Effectiveness of CV towards predicting job performance
Keep Reading
This approach is highly subjective and prone to confirmation bias, where evaluators seek information confirming their pre-existing impressions. The lack of a standardised format for CVs makes consistent and objective assessment extremely difficult, introducing significant inconsistency into the evaluation process.
Perhaps most tellingly, influential meta-analytic research found that core components of the CV — specifically, years of education and amount of work experience – demonstrated very low correlations with subsequent job performance when assessed in their raw form. These correlations were considered so low as to be “unlikely to be useful” for prediction.
Predictive power of CV elements
Looking at specific CV elements, meta-analyses reveal a consistent pattern of weak predictive relationships. Pre-hire work experience (experience applicants bring from previous organisations) shows a corrected correlation of only .06 with subsequent job performance.
When experience is measured simply as duration (years of experience), meta-analyses report low validity coefficients ranging from .13 to .18. Years of education shows a meta-analytic validity of only .10 for predicting job performance.
To put these numbers in context, a correlation of .30 is generally considered moderate, while .50 is strong. The CV components fall well below these thresholds.
Biodata: More promising approach
However, when information about an individual's background, experiences, and accomplishments (similar to that found on a CV) is collected and scored using structured, validated methods through what researchers call “biodata inventories,” it can demonstrate substantially better predictive validity.
Multiple meta-analyses have quantified this relationship. Early reviews estimated the average corrected validity coefficient for biodata predicting overall job performance to be around .32 to .37. More recent meta-analytic work confirms biodata's status as a relatively strong predictor, with operational validity of .35. A comprehensive meta-analysis found that empirically scored composite biodata scales achieved a mean validity of .44 for predicting job performance.
The scoring method matters significantly. Empirically scored biodata composites (where scoring weights are based on statistical relationships with job performance) significantly outperform rationally scored ones (based on expert judgment), with mean validities of .44 versus .24 respectively.
Comparing selection methods
To put the CV's predictive power in perspective, it's useful to compare it with other selection methods. General Mental Ability (GMA) tests show a validity coefficient of .65, while Structured Employment Interviews demonstrate .58. Biodata (Empirically Keyed) ranges from .35 to .44, significantly outperforming Work Experience measured as years (.13-.18), pre-hire experience (.06), and Years of Education (.10). This comparison illustrates the hierarchy of predictive validity.
GMA and structured interviews consistently emerge as top-tier predictors, demonstrating substantially higher correlations with job performance than biodata, and especially higher than simple measures derived from typical CV reviews.
Recommendations for employers
Based on the scientific evidence, organisations seeking to improve their hiring effectiveness should exercise caution with unstructured CV screening, given its low validity and susceptibility to bias.
Where feasible, organisations should prioritise selection methods with stronger meta-analytically supported predictive validity, such as heneral mental ability tests and structured employment interviews.
Systematically developed biodata inventories, particularly those using empirical keying, represent a potentially valid method for leveraging biographical information.
If CVs must be used for initial screening, implementing a structured approach is advisable, involving clear, job-related evaluation criteria based on job analysis and applying them consistently across all candidates.
- Nguwi is an occupational psychologist, data scientist, speaker and managing consultant at Industrial Psychology Consultants (Pvt) Ltd, a management and HR consulting firm. https://www.linkedin.com/in/memorynguwi/ Phone +263 24 248 1 946-48/ 2290 0276, cell number +263 772 356 361 or e-mail: [email protected] or visit ipcconsultants.com.